[FinTech 101] What is fraud? How to use AI to detect a financial fraud for AML?
- FundingReach Holdings Limited
- Dec 4, 2023
- 6 min read
Updated: May 30

Financial fraud poses a significant threat to individuals, businesses, and the stability of the global economy. As fraudulent schemes become increasingly sophisticated and complex, traditional methods of fraud detection struggle to keep pace. However, a transformative force has emerged in the form of Artificial Intelligence (AI) technology, offering innovative solutions to combat financial fraud with unprecedented efficiency and accuracy. In this blog, we will cover more on how to detect financial fraud with AI.
What is fraud?
According to scholar, fraud is defined as cheating, but this understanding has been expanded to cover a wide range of activities. Fraud is a broad term that includes all unintentional, tactical, and devious acts committed by a human being or someone who tries to gain profit from others through false recommendations or coercion of evidence.
Fraud is a deliberate act carried out by one or more officials or people who are not responsible for the regulation, employees, or third parties that relate to the use of fraud to obtain unbalanced or illegal profits. (Diaz, 2013)
Therefore, it can also be understood as any activity that relies on deception in order to achieve a gain. Fraud becomes a crime when it is a “knowing misrepresentation of the truth or concealment of a material fact to induce another to act to his or her detriment” (Black’s Law Dictionary). In other words, if you lie in order to deprive a person or organization of their money or property, you’re committing fraud.
Research also shows that fraudulent activity affecting the financial statement is more prevalent than ever despite statements is more prevalent than ever despite the increased attention devoted to the prevention and detection of fraud by companies and professional accountants. Fraud detection is a critical issue for preparers and users of financial statements, as well as auditor. [1]
Why do people commit fraud?
The Fraud Triangle theory initiated by Donal Cressey Trust violators, when they conceive of themselves as having a financial problem that is non-shareable and have knowledge or wareness that this problem can be secretly resolved by a violation of the position of financial trust. The most widely accepted explanation for why some people commit fraud is known as the Fraud Triangle. The Fraud Triangle was developed by Dr. Donald Cressey, a criminologist whose research on embezzlers produced the term “trust violators.”
The Fraud Triangle hypothesizes that if all three components are present — unshareable financial need, perceived opportunity and rationalization — a person is highly likely to pursue fraudulent activities. As Dr. Cressey explains in the Fraud Examiners Manual: When the trust violators were asked to explain why they refrained from violation of other positions of trust they might have held at previous times, or why they had not violated the subject position at an earlier time, those who had an opinion expressed the equivalent of one or more of the following quotations:
(a) ‘There was no need for it like there was this time.’
(b) ‘The idea never entered my head.’
(c) ‘I thought it was dishonest then, but this time it did not seem dishonest at first.'
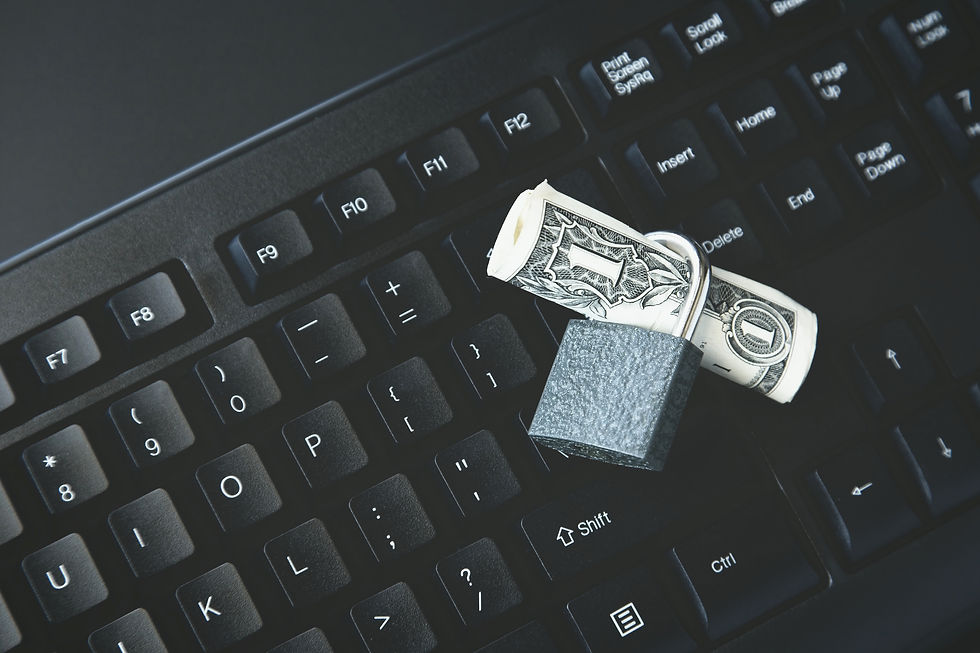
What is the current marketing situation in fraud detection?
According to a market research from the MarketsandMarkets, the global Fraud Detection and Prevention Market size is projected to grow from USD 27.7 billion in 2023 to 66.6 billion by 2028 at a Compound Annual Growth Rate (CAGR) of 19.1% during the forecast period/ The key drivers for market growth are increasing revenue losses and chargebacks due to fraud, heavy usage of digital technologies and IoT across industries, and adopting fraud analytics and risk-based authentication solutions to combat fraud.
One of the main challenges in fraud detection in the market is the lack of trained professionals to analyze fraud attacks. With reference to a survey by the non-profit professional association for Cybersecurity (ISC) revealed that 51% of cybersecurity professionals acknowledged their companies being exposed to significant risks due to the insufficiency of skilled cybersecurity personnel. [2]
Before we talk about how to refine the fraud detection process with AI technology, let us first take a look at how many types of frauds are there.

What are the latest types of fraud in 2023?
With reference to research in 2022, with new types of payments like P2P, come new kinds of fraud such as selling products that do not exist to pocket instant payments. Other types of fraud involve stealing passwords that many users do not properly guard and carelessly manage.
The use of continuous identity proofing is seen as one effective measure against this. This also entails analyzing as many data sources as possible to validate the identity and payment (GIACT, 2020). Another fraud and AML measure is to use machine learning-based artificial intelligence to check the digital identity and perform credit scoring at each POS.
It's not just empty talks.
Let us show you more data here to tell you how essential it is to identify and prevent from fraud detection.
When looking into report payments fraud, according to the current survey on Payments Fraud and Control, conducted by Association for Financial Professionals (AFP), nearly 80% of organizations are most likely to seek assistance from their banking partners for guidance regarding the steps to take to minimize the impact of the fraud and help fraud detection.
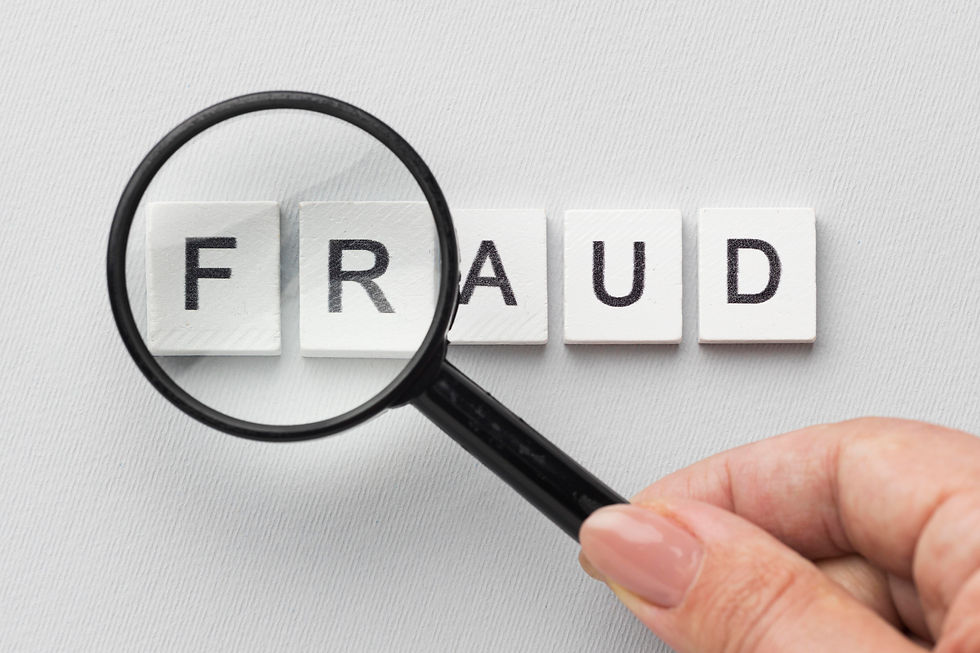
How do institutes apply AI technology into fraud detection in the market?
To prevent fraud and promote financial inclusion, the development of internationally agreed digital-identity standards that will include biometrics is seen as essential. Such a digital identity would be the “golden key” that can access banking services from multiple providers (KPMG, 2019). While this is a robust mechanism, it will weaken the user’s unique security dependence on any one FI.
Existing detection systems depend on defined criteria or learned records which make it difficult to detect new attack patterns. To discover new patterns and achieve higher detection accuracy, machine learning methods based on supervised learning and unsupervised learning and deep learning using artificial neural networks have been actively studied.
Machine learning is one of the key elements in fraud detection. Usually it comes with data reprocessing, sampling, feature selection, application of classification etc. Some scholars also point out the importance of 'deep artificial neural networks' in fraud detection. Deep learning (DL) is a subfield of the machine learning inspired by the structure and function of the brain called artificial neural networks. An artificial intelligence function imitates the working of the human brain in processing data and creating patterns for use in decision-making area, through the capability of unsupervised learning from data that is unstructured or unlabeled. (C. Franc¸ois, 2017)
How to make sure the accuracy in financial fraud detection with AI technology?
Indeed, there are dozens of financial fraud detection methods applying in the market, but one of the common key factors is to make sure that the data base is big enough.
Imbalanced problem in the data could mislead the detection process to the misclassifying problem and a real transaction dataset of financial transaction usually contains a data imbalanced problem. To prevent from the imbalanced problem in the data, various datasets shall be applied to enhance the accuracy Applying wide variety of data shall help generating the different ratio of sampling dataset to increase the reliability and accuracy of our proposed research.[3]
Let’s take iFinGate as an example. iFinGate is well-equipped with over 300 million of data profile, with over 50,000 data sources on its effort acting as clients’ KYC surveillance. iFinGate help scanning data with its one-stop-scan for sanctions, PEP, alert list, and compliance news globally. Together with machine learning on the data base to filter compliance irrelevant data, iFinGate is enabled to provide on-going monitor and provide near real-time update.
To conclude, the integration of AI technology in financial fraud detection is a game-changer, empowering organizations to proactively detect, prevent, and combat financial fraud. By leveraging the potential of AI, we can create a safer and more resilient financial environment, where fraudulent activities are swiftly identified, disrupted, and deterred. To know more about how to apply AI technology in financial fraud detection? Contact us now to tailor a solution!
Read more:
[1] R.T. Edwards, Inc. (2000). What Is Fraud and Who Is Responsible? In Journal of Forensic Accounting Auditing, fraud, and taxation (pp. 247–256). essay.
[2] Fraud detection and prevention market growth drivers & opportunities. MarketsandMarkets. (n.d.). https://www.marketsandmarkets.com/Market-Reports/fraud-detection-prevention-market-1312.html
[3] Choi, D., & Lee, K. (2018). An artificial intelligence approach to financial fraud detection under IOT environment: A survey and implementation. Security and Communication Networks, 2018, 1–15. https://doi.org/10.1155/2018/5483472